Technology Validation
This page aims to answer the question: How do you validate the technology? Ensuring the reliability of our cloud removal models requires rigorous testing and comparison against real-world data. Here, we outline our validation approach, the accuracy metrics we track, and the benchmarks we use to ensure high-quality results.
Validation at Scale
To ensure the reliability of our cloud removal models, we validate them across millions of square kilometers worldwide. Using extensive satellite imagery from diverse climates, landscapes, and seasons, we test how well our models reconstruct cloud-covered areas under real-world conditions. As ClearSKY never interpolates, we can directly validate production data by comparing it to the day before a cloud-free Sentinel-2 validation point.
By leveraging global satellite data, we can systematically compare our outputs against cloud-free reference images, enabling us to measure accuracy across a wide range of environments—from dense forests to open farmland. This large-scale validation ensures that our models generalize well, providing consistently high-quality results regardless of geography or seasonality.
We utilize five years of data to establish robust accuracy benchmarks and monitor for model drift. This allows us to test performance across difficult seasons (e.g. long cloud durations), extreme weather years (e.g. drought years, monsoon seasons), and long-term environmental changes, ensuring that our models remain stable and reliable over time.
Validation Metrics
We use two key metrics to assess our cloud-free reconstructions:
Relative Error (%): This measures how far our predicted values deviate from the correct values. It applies to reflectance values, vegetation indices (such as NDVI), and other spectral properties. For example, if the true reflectance value is 0.1, and our model estimates 0.11, the relative error would be 10%. Lower relative error indicates better accuracy.
Percentage Explained (%): This metric evaluates how well our model captures real-world changes over time. It compares our estimated values against the actual change observed between two cloud-free Sentinel-2 images. For instance, if NDVI increases by 0.1 over 15 days, we assess how much of that change our model accurately reconstructs. This metric provides a strong real-world validation but requires two correctly categorized cloud-free reference images.
Together, these metrics allow us to quantify both absolute accuracy and how well our models preserve meaningful temporal changes. Both metrics requires accurate cloud masking.
Agriculture
For precision agriculture, accuracy is often best evaluated using a vegetation index (e.g., NDVI) rather than raw reflectance values. Ideally, validation should be based on the specific index used in the customer’s application to ensure relevance to their workflow.
In the case above, we identified 17 cloud‐free Sentinel-2 data points between March and November. This allowed us to compute percentage explained for 16 intervals and relative error for all 17 points. Across these 16 intervals, the average percentage explained was 71.5%. Overall, the computed average relative error is 1.12%. As is often the case, long periods show little change, followed by rapid transitions where everything happens at once. Depending on the use case, it may be more relevant to focus on select key intervals, where changes are most critical, to assess accuracy where it truly matters.
Forestry
Forests often remain unchanged for decades, with many reference points showing only minimal variation. This makes traditional accuracy assessments, where all data points are treated equally, less useful, as small fluctuations in stable areas can distort overall error metrics. Instead, event-based validation provides a more meaningful way to assess accuracy by focusing on significant changes, such as deforestation. By emphasizing key events rather than averaging across mostly static points, we ensure that validation reflects the real-world impact of cloud removal on forestry monitoring.
Both BSI (Bare Soil Index) and NDVI (Normalized Difference Vegetation Index) capture a sudden shift when the forest is cleared. NDVI drops sharply, indicating the loss of vegetation, while BSI rises as bare soil is exposed. After the event, a new pattern emerges, reflecting the transition from forest to agriculture. These clear shifts demonstrate how event-based validation can effectively track real-world changes, ensuring that cloud removal preserves critical transformations in land cover.
Denmark Evaluation
While our validation process is applied globally, the following case study from Denmark illustrates our approach in action. We compiled a five‐year stack of satellite images and extracted all cloud‐free instances as validation points, covering urban areas, forests, lakes, and agricultural fields. The statistics presented here, derived from our Stratus-2 model, offer insights into its seasonal performance and help identify potential limitations.
Average MAE: 61.2%
The model captured 61.2% of the mean absolute error on NDVI, indicating the overall discrepancy between predicted and true values.
Change Captured: 68.2%
For periods with over 30 days of cloud cover, 68.2% of the change was captured. This shows how much of the actual change was reflected in our estimates.
Relative Error: 8.78%
The relative error measured on NDVI across all cases was 8.78%, reflecting the proportional error relative to the true values.
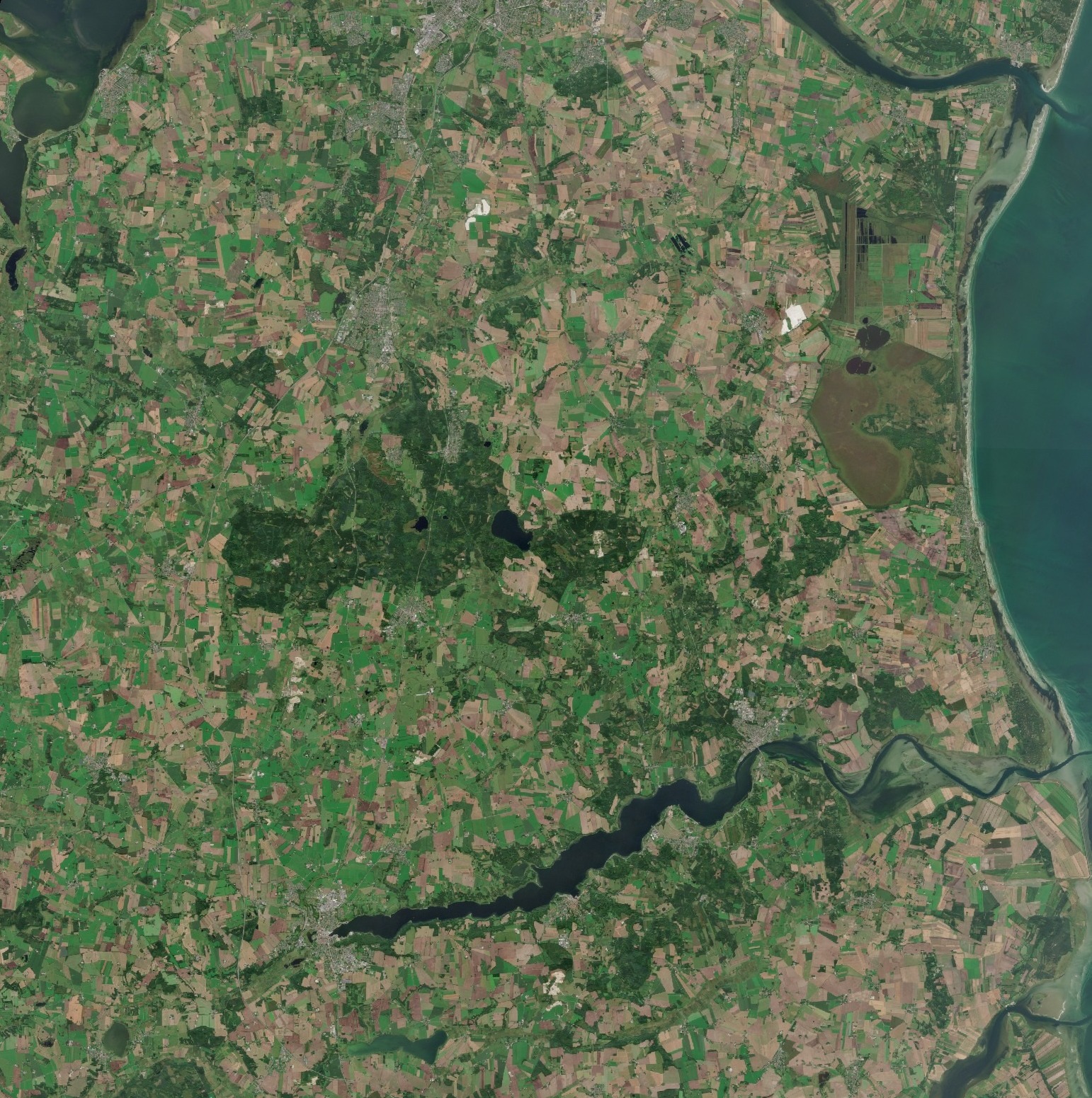